
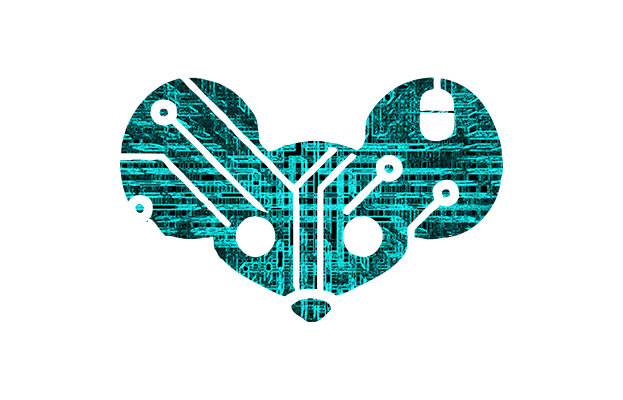
Probably has to be renamed to “ClosedAI” then.
Probably has to be renamed to “ClosedAI” then.
My point is, that the following statement is not entirely correct:
When AI systems ingest copyrighted works, they’re extracting general patterns and concepts […] not copying specific text or images.
One obvious flaw in that sentence is the general statement about AI systems. There are huge differences between different realms of AI. Failing to address those by at least mentioning that briefly, disqualifies the author regarding factual correctness. For example, there are a plethora of non-generative AIs, meaning those, not generating texts, audio or images/videos, but merely operating as a classifier or clustering algorithm for instance, which are - without further modifications - not intended to replicate data similar to its inputs but rather provide insights.
However, I can overlook this as the author might have just not thought about that in the very moment of writing.
Next:
While it is true that transformer models like ChatGPT try to learn patterns, the most likely token for the next possible output in a sequence of contextually coherent data, given the right context it is not unlikely that it may reproduce its training data nearly or even completely identically as I’ve demonstrated before. The less data is available for a specific context to generalise from, the more likely it becomes that the model just replicates its training data. This is in principle fine because this is what such models are designed to do: draw the best possible conclusions from the available data to predict the next output in a sequence. (That’s one of the reasons why they need such an insane amount of data to be trained on.)
This can ultimately lead to occurences of indeed “copying specific texts or images”.
but the fact that you prompted the system to do it seems to kind of dilute this point a bit
It doesn’t matter whether I directly prompted it for it. I set the correct context to achieve this kind of behaviour, because context matters most for transformer models. Directly prompting it do do that was just an easy way of setting the required context. I’ve occasionally observed ChatGPT replicating identical sentences from some (copyright-protected) scientific literature when I used it to get an overview over some specific topic and also had books or papers about that on hand. The latter demonstrates again that transformers become more likely to replicate training data the more “specific” a context becomes, i.e., having significantly less training data available for that context than about others.
When AI systems ingest copyrighted works, they’re extracting general patterns and concepts - the “Bob Dylan-ness” or “Hemingway-ness” - not copying specific text or images.
Okay.
Coding is already dead. Most coders I know spend very little time writing new code.
Oh no, I should probably tell this my whole company and all of their partners. We’re just sitting around getting paid for nothing apparently. I’ve never realised that. /s
While I highly doubt that becoming true for at least a decade, we can already replace CEOs by AI, you know? (:
https://www.independent.co.uk/tech/ai-ceo-artificial-intelligence-b2302091.html
If we’re speaking of transformer models like ChatGPT, BERT or whatever: They don’t have memory at all.
The closest thing that resembles memory is the accepted length of the input sequence combined with the attention mechanism. (If left unmodified though, this will lead to a quadratic increase in computation time the longer that sequence becomes.) And since the attention weights are a learned property, it is in practise probable that earlier tokens of the input sequence get basically ignored the further they lie “in the past”, as they usually do not contribute much to the current context.
“In the past”: Transformers technically “see” the whole input sequence at once. But they are equipped with positional encoding which incorporates spatial and/or temporal ordering into the input sequence (e.g., position of words in a sentence). That way they can model sequential relationships as those found in natural language (sentences), videos, movement trajectories and other kinds of contextually coherent sequences.
Oh yeah, these unrelated autoplay videos are a great pleasure to stop and hide when scrolling. Waste of internet traffic.
There were no ads in the UI of the TV though.
That’s okay. I’m always in for stupid, shitty and flat jokes. It might put a brief smile on someones face and make this existence a little bit more bearable for a second.
I didn’t mean to offend you though.
Thank you. <3
Indeed I was.
I just saw a deleted comment, then your username and found it funny.
Got so angry, that you deleted your own comment, huh? /j
Leave him. He spreads the truth.
What is “inspiration” in your opinion and how would that differ from machine learning algorithms?
From a broad technical perspective “human” “art” is also a process of observing, learning, and recombining to make something new out of it. There is also experimentation which can be incorporated into AI models as well, see for example reinforcement learning, where exploration is an important concept. Therefore, I don’t see how that’s different from “AI” “art”.
However, that should not defend how morally questionable training data is sourced.
They ditched that in 2018. It was long overdue. At least somewhat honest about themselves.
Which exceptions?
They do indeed forbid it.
Deuteronomy 21
Oh man, religions are batshit crazy.